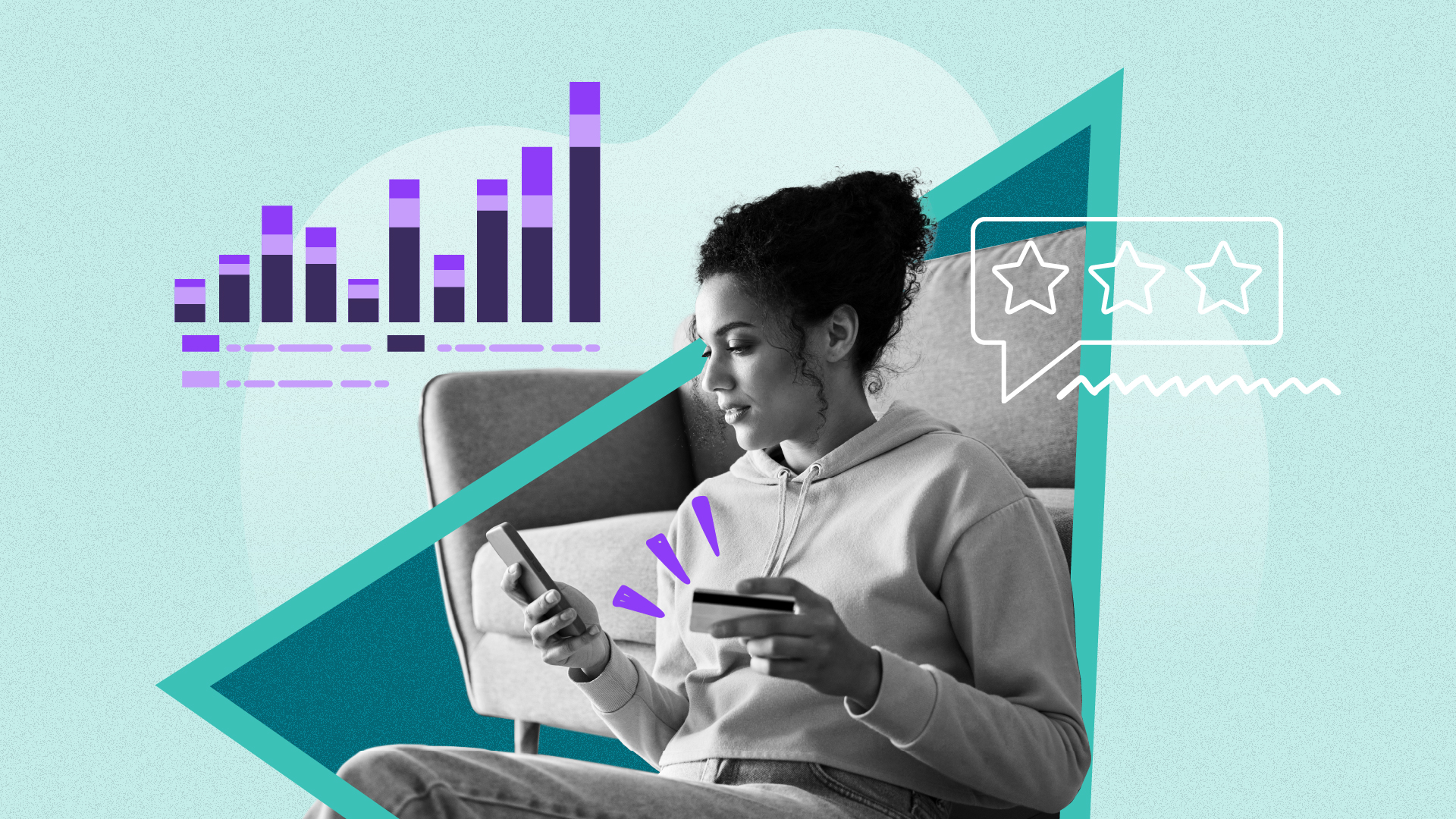
In today’s competitive banking environment, providing exceptional customer experience is crucial. Customer experience management (CXM) strategies are increasingly incorporating process mining to enhance banking services. This blog post explores how process intelligence can improve customer experience management in banking, offering practical insights and answering common questions on the topic.
Process mining analyses should link directly to strategic goals. When used in concert with a process intelligence suite, this can be achieved. In banking, one of the most critical objectives is to improve the customer experience (CX). As you read on, we will outline a practical approach to enhance process mining analysis with CX data to achieve just that.
The challenge: Bridging process and customer perspectives
Pinpointing the customer journey
The first step is to select a critical customer-facing process—such as account opening, security verification, or incident resolution. Collaborate with CX teams (or the team who owns this function) to identify “moments of truth”—those pivotal interactions that significantly impact customer satisfaction. While process mining often focuses on system data, it’s essential to incorporate additional CX-related information to gain a complete picture.
Most banks already run taskforces that have done excellent work mapping customer journeys and identifying pain points. They will have identified the critical touchpoints that determine whether an experience is judged “good” or “bad” via direct feedback.
In CX language, these inflection points are called “moments of truth,” where banks have a chance to impress (or distress) customers. As process miners, we want to understand how to incorporate these touchpoints into our process dataset.
Too often, process mining projects are fixated on system data. While this is where we must start, it should not be where we end. In addition to processing event data itself, it’s crucial to obtain data that exists outside of system records; let’s call this CX-enhancement data. In other words, we model, we mine, and then we enhance.
Defining and combining CX and process data
CX-enhancement data translates qualitative customer feedback into quantitative metrics. For instance, in fraud reporting, defining “good” and “bad” experiences based on response times and resolution outcomes allows us to assign CX scores to each process instance.
By overlaying these scores onto the process execution record, we create a comprehensive dataset that reveals how customer expectations align with actual process performance.
For example, in fraud reporting, customers might provide bad feedback when:
- There is no initial response within 1 hour.
- No resolution within 1 business day.
- And (if needed) no refund was issued within 3 business days.
We need to encode these expectations into our process mining analytical model: Were these expectations met?
The model might look like this:
By adding these criteria to our data model, we reveal previously hidden insights. Although it makes sense that longer response times result in a negative experience, the data has not recorded and graded this. We generate a dataset of moments of truth superimposed over the process execution record. If necessary, we could “weight” these CX ratings to account for more important truth moments.
CX analysis with process mining
With our process dataset enriched with CX metrics, we can now begin to provide insights.
Start by reviewing the execution data with an exploratory analysis. Using the CX scores built into our model, we can create a cohort of cases.
Our objective at this stage is to generate CX-SLAs: through root-cause analysis, determine when cases begin to acquire attributes indicating a poor experience is increasingly likely. Based on the process stage, we highlight instances when the computed “CX score” is below expectations.
This analysis might offer operational teams direction for “remediation windows.” This window is integral between the case’s beginning to exhibit underperformance and reaching a moment of truth. Simply put, there’s still time to mitigate issues.
We can prevent the moment of truth from becoming a painful moment by using our analysis to identify cases within the remediation window and notify operational teams to proactively escalate the case before the customer does. Process mining is now actively contributing to achieving the bank’s CX goals. But we’re not done yet.
Proving the value of process mining
There’s more! We can also generate specific improvement ideas and quantify the expected impact.
With the analysis model, we can create reports on certain case cohorts based on the generated CX score:
- Did-not-breach
- Trended-saved
- Trended-breach
- Intermediate breach
With these cohorts, we can then dig deeper with further analysis and generate recommendations to improve the process.
Several suggestions for CX enhancements:
- Are there specific case types that tend to breach? Can we provide additional training upstream to avoid this?
- Do cases with a poor CX score need more help? Can we recommend a team capacity shift to avoid underperforming activities?
- Can we locate best practices in cases that excel in our CX score checks? Can we share this with other execution teams?
This is all well and good, presumably, because process mining will now be a helpful tool in achieving the strategic objectives of improving CX. However, we will probably face pressure to prove process mining’s financial value creations, particularly in the financial services industry.
We might have helped operational teams focus on critical CX inflection points, but can we quantify the value of this?
Get your CX-focused stakeholders together and discuss the cost of underperformance.
Then, to demonstrate the effectiveness of detection, intervention, and improvement initiatives, we can track the “cost avoidance” of instances where we prevent closed accounts, escalated complaints, and negative reviews.
Furthermore, the model we developed can be used to influence change and illustrate the effects of changes over time. With our findings, we can recommend alterations to the relevant process and change the documented “live” process. In this way, using the power of process mining within a complete process intelligence suite like ARIS, we have the means to continuously improve customer experiences, and then quantify those improvements.
Like process mining, CX enhancement requires teamwork.
It is evident from each stage of this process that cross-functional participation is essential to transformation initiatives. Not only to produce a very influential process mining analysis but also to attain a verified enhancement of the customer experience. It turns out, to no one’s surprise, that achieving strategic goals is difficult and that success requires teamwork.
Where process mining can contribute to customer experience goals in banking is by painting the full picture of the customer’s perspective of actions, going above and beyond reporting the process execution record. We can add current information about how customers interact with process outputs to the dataset, which gives us a case-by-case history of how the typical customer would respond to a certain performance.
The secret is to get vital CX datapoints by involving the appropriate teams inside the bank. Reaching CX objectives can be directly linked to your process mining analysis. You can’t expect process performance indicators to inform you how customers feel about their banking services without this CX data.
Process mining, we’ve seen, can untangle CX knots and get financial services closer to that Amazon experience that customers demand.